Seminar in Psychometrics
The Importance of Latent Variables for Causal Inference
Date and time: Monday, April 14, 2025 (16:00 PM CET)
Place ICS CAS room 318, Pod Vodárenskou věží 2, Prague 8, also on Zoom.
Well-constructed achievement tests are a key strength of educational large-scale assessments (LSAs), and participants’ proficiencies are often the primary focus of subsequent analyses, such as comparisons between different respondent groups. In these non-randomized comparisons, estimating covariate-adjusted group differences is common practice. However, rather than using latent proficiencies directly, researchers often rely on fallible test scores (e.g., sum scores or factor scores) as manifest outcomes or covariates. This talk will highlight the importance of latent variables for causal inference by presenting analytical and empirical results on the impact of measurement error.
First, the theoretical conditions under which latent variables are necessary for causal effect estimation will be outlined, and the bias introduced by measurement error will be derived (Sengewald et al., 2019; Sengewald & Pohl, 2019). Then, various strategies to account for measurement error in causal effect analyses will be summarized, and a recent extension of the R package EffectLiteR for differential effect analysis with latent variables will be introduced (Sengewald & Mayer, 2024). Beyond these theoretical insights, this talk will demonstrate the implementation of different approaches using LSA data from the German National Education Panel Study NEPS; Blossfeld & Roßbach, 2019). Specifically, an analysis evaluating the effectiveness of private tutoring will be presented. The discussion will focus on the practical benefits of using latent variables, the assumptions underlying different analysis approaches, and future developments.
References:Blossfeld, H. P., & Roßbach, H. G. (Eds.). (2019). Education as a lifelong process: The German national educational panel study (NEPS). Edition ZfE (2nd ed.). Springer VS.
Sengewald, M.-A., & Mayer, A. (2024). Causal effect analysis in nonrandomized data with latent variables and categorical indicators: The implementation and benefits of EffectLiteR. Psychological Method, 29(2), 287–307. https://doi.org/10.1037/met0000489
Sengewald, M.-A. & Pohl, S. (2019). Compensation and amplification of attenuation bias in causal effect estimates. Psychometrika, 84(2), 589-610. https://doi.org/10.1007/s11336-019-09665-6
Sengewald, M.-A., Steiner, P. M., & Pohl, S. (2019). When does measurement error in covariates impact causal effect estimates? - Analytical derivations of different scenarios and an empirical illustration. British Journal of Mathematical and Statistical Psychology, 72(2), 244-270. https://doi.org/10.1111/bmsp.12146
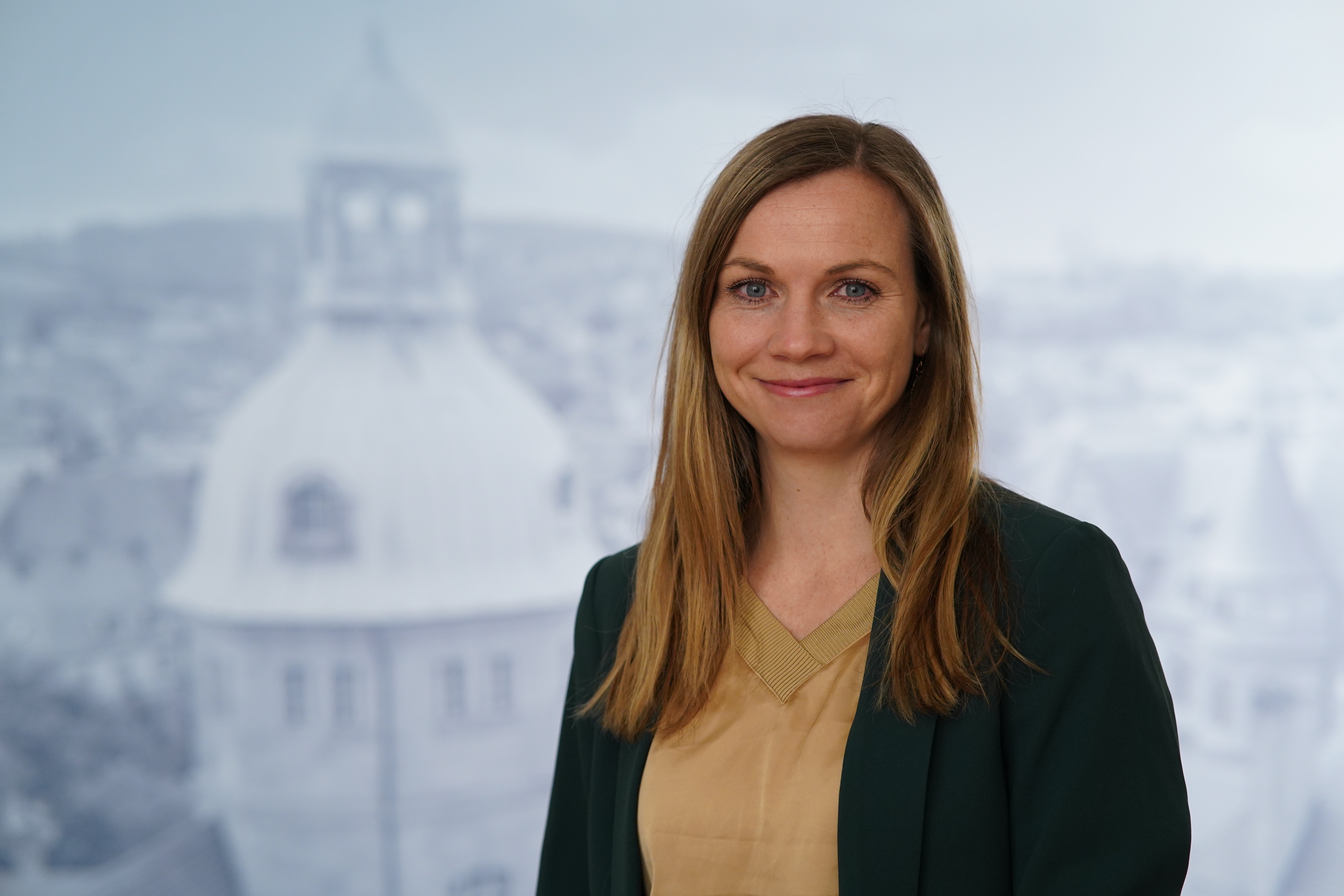
Marie-Ann Sengewald
Dr. Marie-Ann Sengewald is a postdoctoral researcher specializing in methods development at the Leibniz Institute for Educational Trajectories (LIfBi) in Germany. Her work focuses on quantitative practices in psychometrics, causal inference, and metascience, with a particular interest in the interrelation of these research fields. She earned her Diploma in Psychology from Friedrich Schiller University Jena in 2011 and completed her doctorate at Freie Universität Berlin in 2019, with a dissertation on Latent Covariates. Dr. Sengewald has held research and teaching positions at Friedrich Schiller University Jena and Otto Friedrich University Bamberg. Since 2019, she has been a research associate at LIfBi, where she currently leads the DFG-funded project Conceptual Replications. Her contributions to the field have been recognized with several honors, including the Marie-Schlei-Preis 2020 from Freie Universität Berlin for her dissertation and the Sigrid Blömeke Scholarship Award 2025 from CREATE - Center of Excellence for Research on Equality in Education at the University of Oslo.