Seminar in Psychometrics
Identifiability in graphical models with algebraic methods
Date and time: November 28, 2024 (1:00 PM CET)
Place ICS CAS room 318, Pod Vodárenskou věží 2, Prague 8, also on Zoom.
Graphical models provide a tractable framework to encode stochastic dependencies between a set of random variables. A typical family are the linear structural equation models (linSEMs) in which every edge is associated with a linear coefficient. The cognitive diagnosis models (CDMs) are special bipartite graphical models with Bernoulli distribution parameters, which aim to infer individuals' latent attributes based on their observed responses to a set of designed diagnostic item. The CDMs are widely used in psychometrics for individual diagnosis and targeted treatment.
Identifiability is an essential topic for statistical models and conditions under which the models are identifiable can guide data collection and test design. In this presentation, I will introduce some algebraic methods for different notions of identifiability in parameterized models. The application of those methods and derived results in linear structural equation models and cognitive diagnostic models will be discussed.
References:Mathias Drton, Benjamin Hollering, and Jun Wu (2025). Identifiability of homoscedastic linear structural equation models using algebraic matroids. Advances in Applied Mathematics, 163, 102794. https://doi.org/10.1016/j.aam.2024.102794
Iván Pérez and Jiří Vomlel (2023). On identifiability of BN2A networks. In European Conference on Symbolic and Quantitative Approaches with Uncertainty, 136–148. Springer. https://doi.org/10.1007/978-3-031-45608-4_11
Seth Sullivant. (2018). Algebraic statistics, volume 194. American Mathematical Soc. https://doi.org/10.1090/gsm/194
Marloes Maathuis, Mathias Drton, Steffen Lauritzen, and Martin Wainwright (2018). Handbook of graphical models. CRC Press. https://doi.org/10.1201/9780429463976
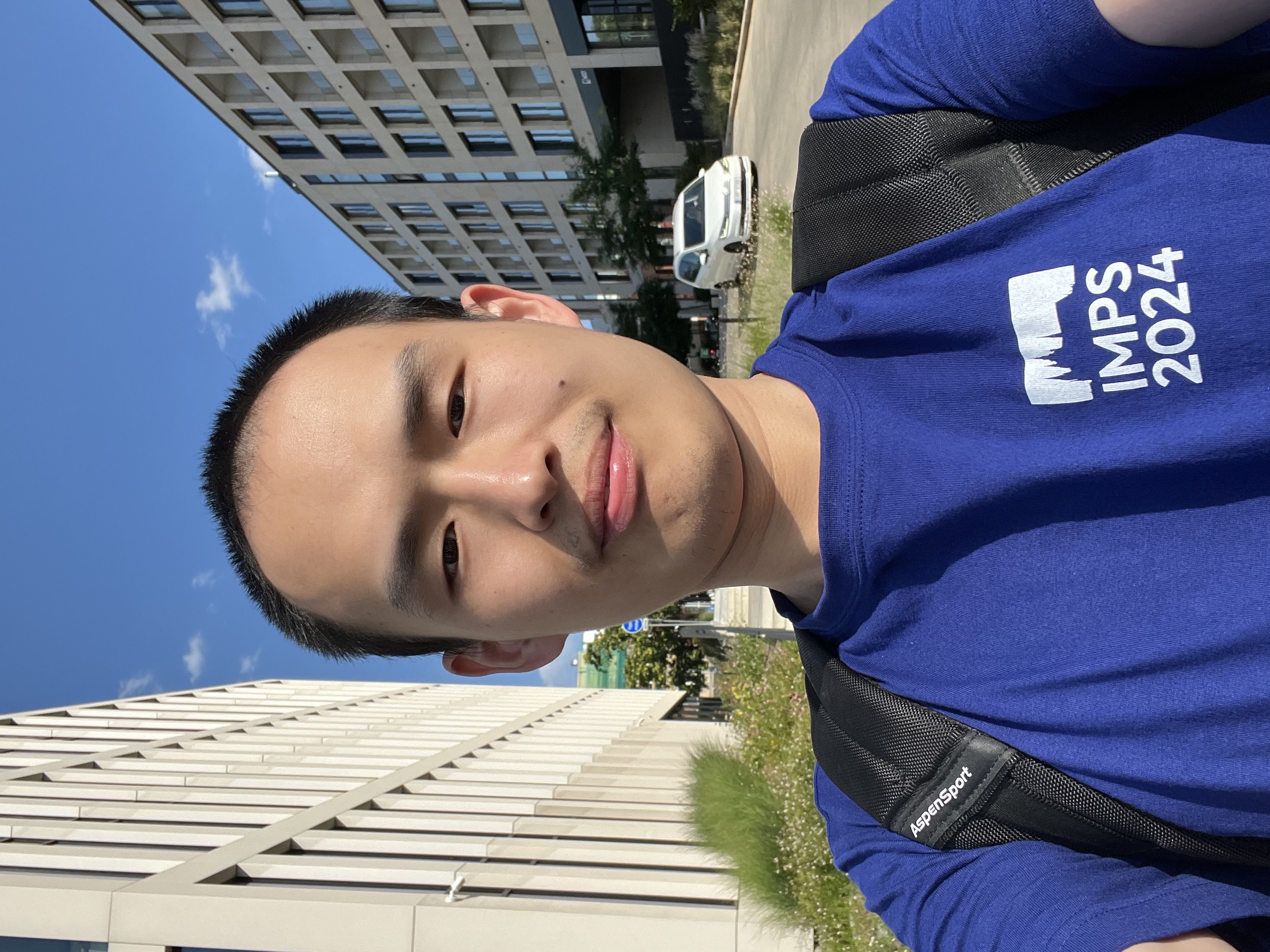
Jun Wu
Jun Wu obtained his doctoral degree in the Chair of Mathematical Statistics (Prof. Mathias Drton) at Technical University of Munich. His research focuses on causality, graphical models, and algebraic statistics. He will soon begin a postdoctoral position at the Institute of Computer Science, Czech Academy of Sciences, where he will work on properties and estimation algorithms for cognitive diagnostic models.