Seminar in Psychometrics
Computational Psychometrics in Action: Calibrating & Administering New Items in a CAT
Date and time: Monday, May 5, 2025 (16:00 PM CET)
Place On Zoom, streamed to ICS CAS room 318, Pod Vodárenskou věží 2, Prague 8.
In this presentation, I describe a new system for efficiently calibrating and administering a large-scale computerized adaptive test (CAT) with new items with a small number of responses. This work has been conducted by the interdisciplinary R& D team from the Duolingo English Test. Calibration — learning item parameters in a test — is done here using AutoIRT, a new method that uses automated machine learning (AutoML) in combination with item response theory (IRT), originally proposed in Sharpnack et al (2024). AutoIRT trains a non-parametric AutoML grading model using item features, followed by an item-specific parametric model, which results in an explanatory IRT model. Item selection in a CAT is done here through an adaptive selection algorithm called BanditCAT, and an extension for the item selection called S2A3. These approaches were developed by casting the adaptive administration in the contextual bandit framework and Bayesian Item Response Theory (IRT). Contextual bandits are machine learning (ML) methods that use a reinforcement learning approach for sequential decision making, and have been used in recommender systems across industries. As an extension of this, Soft-Scoring and Adaptive Adaptive Administration (S2A3) is introduced, which accounts for uncertainty in item parameter estimates and enables periodic item re-calibration. This methodology enables the scoring and administration of items for which we have little to no response data. This work bridges the gap between ML approaches to sequential decision making and psychometrics for adaptive testing, hence computational psychometrics (von Davier et al, 2022). The methods are illustrated with data from the Duolingo English Test (DET)'s practice test.
References:Lockwood, J. R., & Nydick, S. W. (2023, July). Scalable explanatory IRT modeling with sparse data structures. Paper presented at the International Meeting of the Psychometric Society, College Park, MD, USA.
Sharpnack, J., Hao, K., Mulcaire, P., Bicknell, K., LaFlair, G., Yancey, K. & von Davier, A.A.. (2025). BanditCAT and AutoIRT: Machine learning approaches to computerized adaptive testing and item calibration. Large Foundation Models for Educational Assessment. PMLR. https://proceedings.mlr.press/v264/sharpnack25a.html
Sharpnack, J., et al. (2024, September). A Thompson sampling approach to IRT-based computerized adaptive tests. Paper presented at the International Association of Computerized Adaptive Testing, Seoul, Korea.
von Davier, A. A., Mislevy, R. J., & Hao, J. (Eds.). (2021). Computational psychometrics: New methodologies for a new generation of digital learning and assessment: With examples in R and Python. Springer International Publishing. https://doi.org/10.1007/978-3-030-74394-9
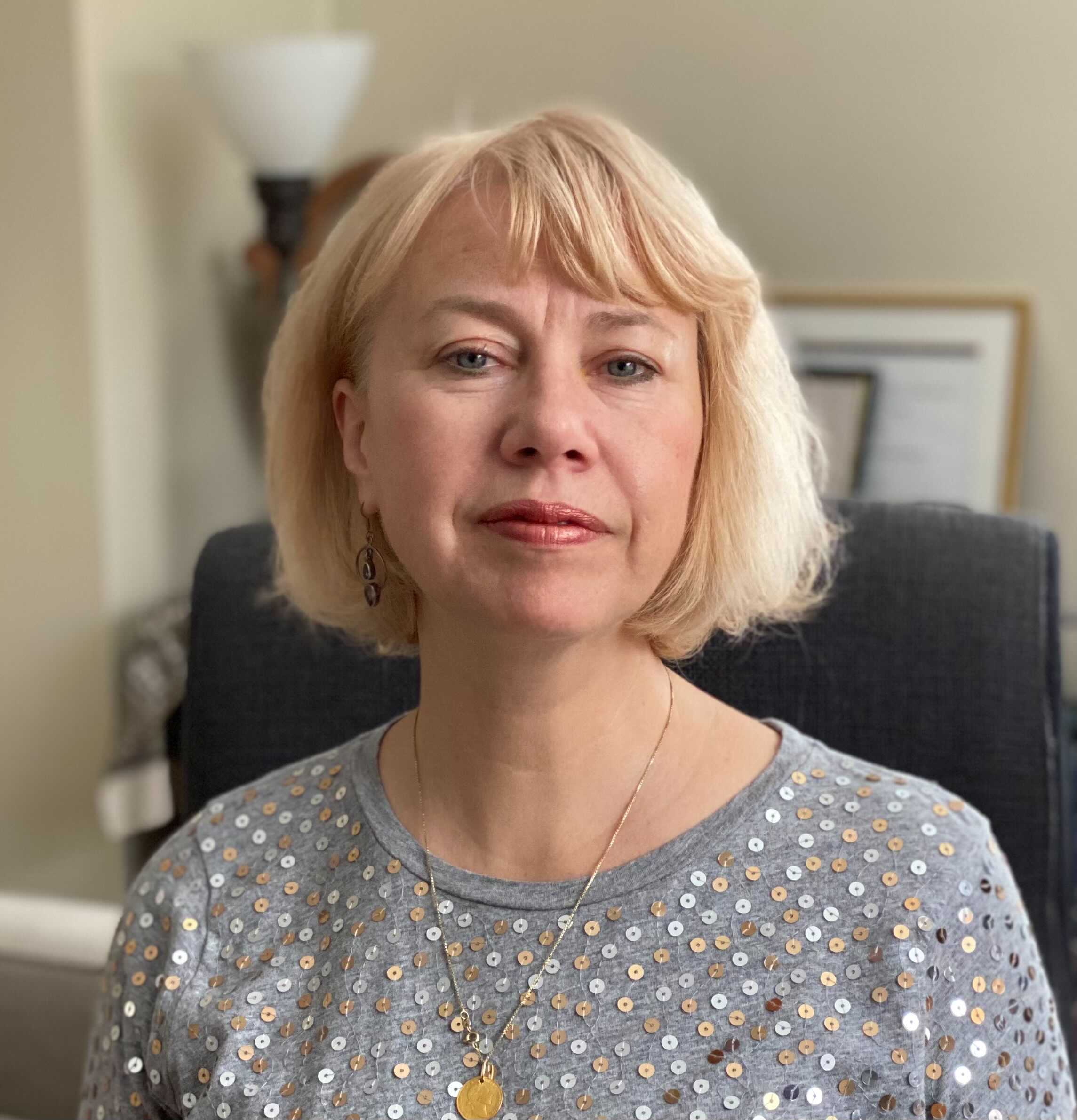
Alina von Davier
https://en.wikipedia.org/wiki/Alina_von_Davier
Alina A. von Davier is a researcher, innovator, and an executive leader with over 20 years of experience in EdTech and in the assessment industry. She is currently the Chief of Assessment at Duolingo, where she leads the Duolingo English Test research and development area. She is also the Founder and CEO of EdAstra Tech, a service-oriented EdTech company. In 2022, she joined the University of Oxford as an Honorary Research Fellow, and Carnegie Mellon University as a Senior Research Fellow. She currently serves as a Non-executive Director on the Board for MACAT, an EdTech company focused on critical and creative thinking, learning and assessment and she is a Venture Partner for LearnLaunch Fund and Accelerator. Her research is in the field of computational psychometrics, machine learning, and education. Von Davier was a Chief Officer at ACT, where she led ACTNext, an innovation unit. Previously she was a Senior Research Center Director at Educational Testing Service. Von Davier earned an M.S. in Mathematics from the University of Bucharest in 1990, and a Doctorate in Mathematics from Otto von Guericke University Magdeburg in 2000. She also completed classes in an Executive MBA program from Harvard Business School in 2019. Von Davier’s work has been widely recognized in the academic community. In 2019, she was a finalist for the Innovator award from the EdTech Digest. In 2020, she received ATP’s Career Award for her contributions to assessment. The American Educational Research Association awarded her the Division D Signification Contribution Educational Measurement and Research Methodology Award for her publications “Computerized Multistage Testing: Theory and Applications” (2014) and an edited volume on test equating, “Statistical Models for Test Equating, Scaling, and Linking” (2011). In 2022 she also received the Brad Hanson award from National Council in Education (NCME) for her work on adaptive testing and for the co-authored book on computer adaptive testing with R. See von Davier’s GoogleScholar page.